Table of contents
Chapter 1 First Steps
You learn how this volume differs from other introductions to multilevel modeling. Throughout, only one small dataset and one outcome are examined. You already know these data. You can check the recommended qualifications to see if you are ready to start mastering multilevel models. The short version is this. You want to read this book if you work with quantitative data that are clustered spatially, organizationally, or temporally. You are ready to read this book if you have a basic understanding of monolevel statistics like analysis of variance and multiple regression, as well as access to the Stata statistical program. Upcoming topics in other chapters are mapped.
​
Chapter 2 From Title Deeds to Dataset
This chapter explains how information on the front side of each of the 22 Monopoly® Title Deed cards has been turned into a dataset. For each property, its sequence along the board, its color, and its position on one of the four different sides of the playing board can function as an indicator of that property’s location-linked relative socioeconomic status (SES) in Atlantic City, New Jersey, in the first half of the 20th century. A 1910 aerial map, with Mediterranean Avenue and the Boardwalk singled out, illustrates the geographic variation driving neighborhood socioeconomic status (SES) differences. The game’s designers incorporated these SES differences into several game features.​
​
Chapter 3 Describing the Dataset Statistically
When starting with a new dataset, get to know it before jumping in and doing analyses. Stata commands like summarize, describe, and codebook, provide an initial overview. Generating a table of descriptive statistics for the outcomes and predictors used in your analysis – and looking carefully at the information displayed – is an essential second step. This chapter describes how to generate such a table.
​
Chapter 4 Monolevel Models Part I: OLS (Ordinary Least Squares) Regression
Following the recommendation to run monolevel models before multilevel models, this chapter runs ordinary least squares (OLS) bivariate regression models using three alternate socioeconomic status (SES) variables to predict rent. Results across three models are compared. b weights, constants, and R2 values are interpreted. Blalock’s (1979) suggestion for interpreting significance tests when working with population data is explained.
​
Chapter 5 Monolevel Models Part II: Oneway Analysis of Variance (ANOVA)
Each of the three SES variables used in Chapter 4 to predict rent may not have fully captured all between-group differences in rent values. In contrast, a oneway ANOVA model does analyze group-level rent differences in their entirety. This chapter displays oneway ANOVA results, examines pairwise significant group differences in rent using post-hoc tests, and uses a water buckets analogy to explain what is happening.​
​
Chapter 6 First Multilevel Model: No Predictors
The multilevel model with no predictors, also called the ANOVA model or the null model, estimates how much outcome variation resides at each level of the model. Rather than a model with one intercept for all groups, here the model allows each group its own estimated mean score on the outcome. These estimates are made following empirical Bayes adjustments to group-level outcome means, and can be considered latent variables. Results include both descriptive information and a statistical test of the significance of group-level mean outcome differences. Post-hoc caterpillar plots reveal which pairs of groups have significantly different outcome means.​
​
Chapter 7 Second Multilevel Model: Adding Guesthouses and Hotels
Two level-1 predictors enter the model: the number of guesthouses, and the presence or absence of a hotel. In this analysis of covariance (ANCOVA) model attention can center either on the impact of these specific predictors, or on remaining neighborhood differences after including these variables. Resulting output is unpacked line by line. Two key questions merit investigation. (1) What does it take to completely control for level-2 neighborhood differences? The difference between treating neighborhood differences as random effects versus fixed effects is explained. (2) What are the different ways to think about R2 in a multilevel model? One recommended approach is reviewed.​
​
Chapter 8 Third Multilevel Model: Do Neighborhoods Alter Hotel Rent Impacts?
Are hotel rent impacts stronger or weaker, depending on the neighborhood? This question drives the models examined in this chapter. Multilevel models where impacts of a level-1 predictor may depend on level-2 context embody cross-level interactions and are called random coefficient regression models. To learn whether hotel rent impacts indeed are context dependent, a seven step investigatory process is outlined, a series of models are formulated, and results from different models are compared. Close scrutiny reveals a serious theoretical flaw with the model whose predictions align most closely with the data. The chapter serves as a cautionary tale while simultaneously detailing the key theoretical and operational steps involved in formulating, testing, and examining a model with a cross-level interaction. ​
​
Chapter 9 Longitudinal Data: Did Some Properties Develop Faster than Others?
Multilevel models provide a powerful tool for analyzing longitudinal data. Each stage of real estate development for each property is tied to a hypothetical year between 1880 and 1930. A random coefficients regression model allowing the impact of hypothetical year to vary by property considers whether each property charted its own development pathway through time. Stated differently, did all the properties reveal the same predicted impact of each passing year on increasing rent? Or, alternatively, did the predicted rent impact of each passing year vary significantly across the 22 properties? The investigation follows the seven steps used in the previous chapter. Different property-level predicted development trends mimic historical geographic differences in rates of development. As in the previous chapter, careful checking reveals a serious theoretical problem with the preferred model, providing another cautionary tale. Topics of serial autocorrelation and linear versus curvilinear impacts are introduced.​
​
Chapter 10 Centering and Multileveling Predictors
This chapter tackles two matters: centering and multileveling. Worked examples use mathematicians Abbott & Richey’s (1997) landing probability variable, the chances of landing on each space on the Monopoly ® playing board. Grand mean centering a predictor removes the overall mean from each value. Group mean centering removes the respective group mean from each level-1 value, creating an indicator for a new concept that reflects frog pond effects. Group mean centered level-1 predictors, however, cannot control for compositional effects. Turning to the second topic, of course multilevel models can include predictors captured at level 2. Level-1 predictor impacts on an outcome, however, might reflect both between-group and within-group dynamics. A multileveled predictor separates those dynamics. The multileveled predictor includes group-mean centered values at level 1, and group mean values at level 2. Whether group mean centering a predictor, or multileveling a level-1 predictor, be clear about the corresponding theoretical rationales.​
​
Chapter 11 Next Steps
Chapter suggests additional sources for learning more about multilevel models.
​​
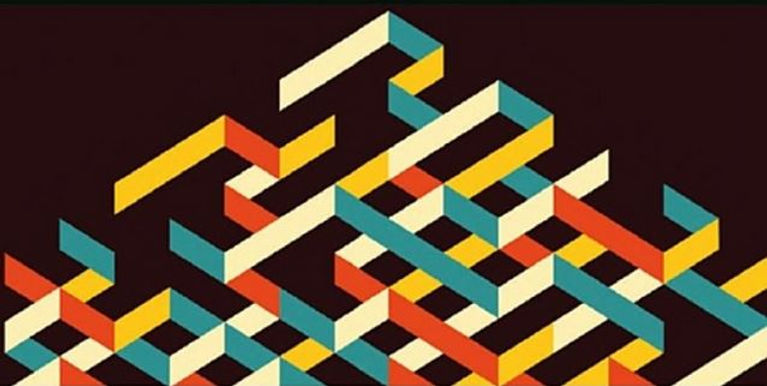